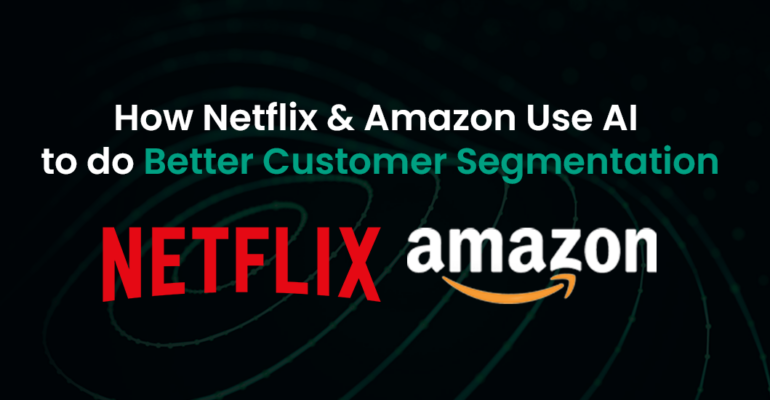
By Rahul Pandita
14th July, 2024
Netflix uses AI to enhance customer segmentation through a multifaceted approach, leveraging advanced algorithms and vast amounts of data to understand and predict user preferences and behaviors. Here’s a detailed look at how Netflix uses AI to achieve superior customer segmentation:
Personalized Content Recommendations
Netflix’s recommendation system is one of the most sophisticated examples of AI-driven customer segmentation.
The system uses collaborative filtering, content-based filtering, and deep learning algorithms to analyze viewing habits, ratings, and behavior patterns of millions of users.
Collaborative filtering identifies patterns by examining similarities between users and items, while content-based filtering focuses on the attributes of the content itself.
Algorithms Used
Matrix Factorization
Helps in identifying latent factors in the data that influence user preferences.
Recurrent Neural Networks (RNNs)
Used for sequential data to understand the order in which users watch content.
Restricted Boltzmann Machines (RBMs)
Employed for learning the hidden representations of user-item interactions.
Dynamic User Profiles
Netflix constantly updates user profiles in real-time. As users watch more content and interact with the platform, the AI algorithms continuously refine and adjust the segmentation. This ensures that recommendations remain relevant and personalized.
Techniques Used
Real-Time Data Processing
Incorporates new viewing data immediately to keep profiles current.
Event Stream Processing
Uses tools like Apache Kafka to handle the influx of real-time data and update models accordingly.
Behavioral and Psychographic Segmentation
Beyond basic demographic data, Netflix delves into behavioral and psychographic segmentation. The platform analyzes detailed behavior patterns, such as the time of day users watch content, binge-watching habits, and genre preferences.
Viewing Time and Duration
Tracks when users are most active and for how long.
Interaction Data
Analyzes how users interact with the interface, such as pause, rewind, and fast-forward actions.
Social Signals
Incorporates social media data and user-generated content to understand trends and preferences.
A/B Testing and Continuous Improvement
Netflix employs extensive A/B testing to experiment with different algorithms and recommendation strategies. This iterative process helps fine-tune their AI models for better segmentation.
A/B Testing Process
Hypothesis Formulation
Develops hypotheses on how changes in algorithms might improve segmentation and user engagement.
Experimentation
Runs controlled experiments with different user groups to test these hypotheses.
Analysis
Uses statistical methods to analyze the results and determine the most effective strategies.
Content Creation and Acquisition
AI-driven segmentation also informs Netflix’s content creation and acquisition strategies. By understanding what different segments prefer, Netflix can invest in producing or acquiring content that aligns with the tastes of its diverse user base.
Data-Driven Decisions: Genre Popularity
Identifies which genres are trending among different user segments.
Content Gaps: Detects unmet needs and preferences that can be targeted with new content. Regional Preferences: Analyzes geographic data to cater to local tastes and preferences.
Enhanced Customer Retention AI helps Netflix maintain high customer retention rates by keeping the platform engaging and relevant. Personalized recommendations and timely content updates reduce churn by continually offering value to users.
Retention Strategies: Churn Prediction Models
Uses machine learning to predict which users are likely to unsubscribe and deploys targeted retention campaigns.
Engagement Metrics: Monitors user engagement metrics to identify at-risk segments and intervene with personalized content and offers.
User Interface Personalization
Netflix personalizes the user interface itself based on segmentation data. This includes customized thumbnails, personalized rows, and tailored content carousels to make the browsing experience more engaging.
Interface Customization
Thumbnails
Dynamically changes thumbnails based on what a user is likely to click on.
Content Rows
Orders and organizes content rows based on predicted user interest.
Search and Navigation
Tailors search results and navigation paths to individual user preferences.
Ethical Considerations and Data Privacy
Netflix ensures ethical use of AI and data privacy by adhering to strict guidelines and regulations. The company is transparent about its data usage policies and employs robust security measures to protect user data.
Transparency
Clearly communicates how user data is collected and used.
User Control
Provides users with options to manage their data and preferences.
Data Security
Implements advanced encryption and security protocols to safeguard user information.
How Amazon Uses AI for Customer Segmentation
Amazon uses AI to significantly enhance customer segmentation, leveraging advanced technologies to provide personalized experiences, improve customer engagement, and drive sales. Here is a detailed exploration of how Amazon employs AI for better customer segmentation:
Personalized Product Recommendations
Amazon’s recommendation system is a cornerstone of its AI strategy, employing collaborative filtering and deep learning techniques.
The recommendations are displayed on various interfaces, such as the Amazon homepage, product detail pages, and personalized emails.
This system analyzes each customer’s purchase history and behavior to match them with products they are likely to buy.
The recommendations are displayed on various interfaces, such as the Amazon homepage, product detail pages, and personalized emails.
This personalization drives a significant portion of Amazon’s sales, as it increases the relevance of the products shown to each customer.
Amazon Personalize
Amazon Personalize is a machine learning service that enables developers to create individualized recommendations for users.
It allows for intelligent user segmentation by learning from user interactions and preferences. This service can be used to tailor content across various channels, including websites, mobile apps, emails, and advertisements.
Amazon Personalize leverages data such as user clicks, views, and purchase history to generate highly accurate segments and recommendations, enhancing user engagement and conversion rates.
Real-Time Personalization
For instance, as users browse products, Amazon’s algorithms analyze their behavior in real-time to update recommendations dynamically.
Amazon employs real-time personalization techniques to ensure that the content shown to users is immediately relevant.
This approach keeps the shopping experience fresh and tailored to the user’s current interests and needs.
Voice Shopping with Alexa Amazon’s voice assistant
For example, Amazon Personalize can generate personalized subject lines for emails, enhancing open rates and engagement. By analyzing customer data, generative AI can craft messages that resonate more effectively with specific segments, making marketing efforts more efficient and impactful.
Through voice shopping, users can add items to their shopping lists, reorder products, and receive personalized product suggestions.
Alexa, is integrated into its e-commerce platform to offer a seamless shopping experience. Alexa uses natural language processing and machine learning to understand user commands and preferences.
This hands-free shopping method enhances convenience and further personalizes the customer experience by leveraging past purchase data and user preferences.
Generative AI for Marketing Amazon uses generative AI to create compelling content for marketing campaigns.
Behavioral and Psychographic Segmentation
Beyond demographic data, Amazon delves into behavioral and psychographic segmentation to understand deeper customer motivations. This includes analyzing browsing patterns, purchase frequency, and even time spent on different parts of the website. Such detailed segmentation allows Amazon to target customers with highly specific interests and behaviors, resulting in more effective marketing strategies.
Improving Customer Retention
AI-driven customer segmentation also plays a pivotal role in improving customer retention. By understanding which segments are at risk of churn, Amazon can deploy targeted retention strategies. These might include personalized offers, tailored communications, and proactive customer service interventions designed to keep customers engaged and satisfied.
Amazon SageMaker
Amazon SageMaker is another critical tool in Amazon’s AI arsenal for customer segmentation. It allows businesses to build, train, and deploy machine learning models at scale.
SageMaker can process unstructured data, such as customer reviews and social media interactions, to uncover hidden patterns and insights.
This capability is crucial for creating sophisticated customer segments that reflect real-world behaviors and preferences.
Customer segmentation is the process of dividing a company’s customers into distinct groups based on specific characteristics.
Segmentation criteria can include demographic factors (age, gender, income), geographic location, psychographic traits (lifestyle, values), and behavioral aspects (purchase history, brand loyalty).
This practice allows businesses to tailor their marketing, sales, and product strategies to meet the unique needs of each segment.
AI for Better Customer Segmentation?
AI holds immense potential in transforming customer segmentation, fundamentally changing how businesses understand and interact with their customers.
One of the most significant advantages of AI is its precision and accuracy. By analyzing vast datasets, AI can identify patterns and insights that traditional methods often miss.
This allows for more accurate and granular segmentation, giving businesses a deeper understanding of the nuanced differences between customer groups.
Moreover, AI enables real-time analysis, allowing businesses to respond quickly to changing customer behaviors and market conditions.
This agility ensures that marketing and sales strategies remain relevant and effective. For instance, with predictive analytics powered by AI, businesses can forecast future customer behaviors and trends.
This foresight enables them to proactively tailor their offerings, improving customer satisfaction and loyalty.
Another transformative aspect of AI in customer segmentation is its ability to deliver personalization at scale.
AI can create hyper-personalized experiences for individual customers based on their specific behaviors and preferences.
This capability significantly enhances customer engagement and retention rates. By delving deep into customer behavior, AI analyzes factors such as purchase history, browsing patterns, and social media interactions. These insights help businesses understand what drives customer decisions, enabling more effective targeting and messaging.
Efficiency and cost savings are also crucial benefits of AI-driven segmentation. Automating the segmentation process reduces the time and resources needed for data analysis, allowing businesses to allocate their resources more effectively towards strategy and execution.
Additionally, AI enhances customer journey mapping by identifying key touchpoints and interactions that influence purchasing decisions. This holistic view helps businesses optimize every stage of the customer experience.
AI’s dynamic nature is another strength. It continuously updates and refines customer segments based on new data and insights, ensuring that segmentation remains current and relevant as customer behaviors evolve.