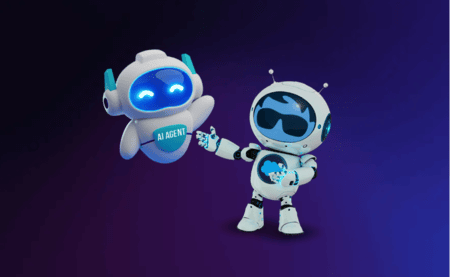
From Agents to Action: Inside Salesforce’s Agentforce and the Future of Autonomous AI in the Enterprise
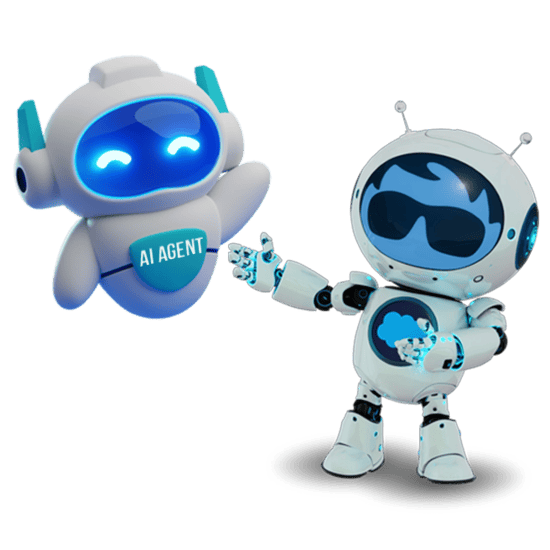
By Teqfocus Team
17th April, 2025
Welcome to a behind-the-scenes look at the evolution of Agentic AI, straight from the minds building it. In this episode of TeqTalk, we sat down with Manjeet Singh – AI expert, fitness coach, and a key product leader at Salesforce – to unpack what’s happening under the hood of Agentforce, Salesforce’s multi-agent orchestration platform.
If you’re a C-suite executive, product strategist, or innovation leader evaluating AI implementation, this conversation is your blueprint for the next frontier of enterprise productivity.
Identity in the Age of AI and Fitness
Manjeet’s dual identity – AI technologist and disciplined fitness practitioner – sets the tone for the discussion. Just like AI agents require structure, training, and feedback loops to perform, the human body thrives on the same principles. This metaphor remains central throughout the episode, offering a unique lens on the discipline and engineering behind AI transformation.
From Chatbots to Agents: Why It’s Not Just a Fancy Rename
A common misconception is that AI agents are just glorified chatbots. But as Manjeet outlines, they’re fundamentally different in design and execution. While chatbots respond to prompts within narrow rules, AI agents are autonomous executors – capable of understanding preferences, pulling data from multiple systems, and adapting over time.
“If a chatbot helps you find flights, an AI agent compares preferences, checks live pricing, and autonomously rebooks when prices drop.”
McKinsey calls these “cognitive agents” and estimates they could automate up to 70% of business activities across sectors by 2030.
The Evolution: Chatbots → Copilots → Agents
Agentforce represents the natural progression from basic automation to true AI orchestration. This maturity curve is important:
- Chatbots: Rule-based and reactive
- Copilots: Embedded, suggestive, task-focused
- Agents: Contextual, proactive, goal-oriented
According to Gartner, by 2026, over 60% of customer service interactions will be handled by autonomous agents – up from 10% in 2021.
What Makes an AI Agent, Really?
Manjeet breaks down an AI agent into four building blocks;
- Model – The core reasoning engine (e.g., OpenAI, Salesforce’s proprietary models)
- Instructions & Guardrails – What the agent can and can’t do
- Memory – Persistent context across interactions
- Data & Integration Layer – Access to enterprise systems for grounded responses
Forrester emphasizes that “contextual grounding” is essential to reduce hallucinations in enterprise AI.
Agentforce = Platform, Not Just a Feature
Agentforce isn’t just a single tool – it’s a low-code/no-code platform enabling organizations to build and deploy agents specific to service, sales, or marketing workflows.
Salesforce’s ecosystem supercharges Agentforce with;
- MuleSoft: API-based integration
- Data Cloud: Customer 360 and unified data
- Einstein AI: Native intelligence for predictive actions
90% of firms using integrated platforms like Salesforce+MuleSoft have reported faster go-to-market execution.
The Future is Multi-Agent
Imagine a Service Agent autonomously handing off a case to a Sales Agent who checks inventory and consults a Compliance Agent before offering a discount – all in seconds. That’s the future Salesforce is building.
While cross-agent handoffs are evolving, the groundwork is being laid. Interoperability, metadata exchange, and collaborative protocols between agents – even from different vendors like OpenAI and Google – are actively in development.
“The real unlock is enterprise agents talking to consumer agents.”
Harvard Business Review calls this “agent ecosystems” – where different AI roles collaborate to simulate human teamwork.
The Memory Gap in Enterprise AI
A key challenge in AI agent orchestration is persistent memory. Most large language models lack native long-term memory, requiring additional tooling.
Salesforce’s solution? The Atlas Versioning Engine – a context management and memory orchestration layer designed for CRM use cases. It retains task context, enables long conversations, and determines when to “forget” based on timeouts or intent switches.
This mimics cognitive science principles, echoing Daniel Kahneman’s “System 1 vs. System 2” thinking -fast vs. slow cognition.
Agentforce vs. RPA & Workflow Automation
Traditional automation (RPA, decision trees) is static and deterministic. Agentforce introduces adaptive automation that;
- Handles ambiguous inputs
- Evolves based on feedback
- Executes multiple-step goals without predefined flows
For example, where RPA might process a refund request, an AI agent could evaluate refund eligibility, suggest alternatives, update the CRM, and notify the customer – all without human input.
According to Deloitte, intelligent automation can deliver up to 30–50% cost savings in operational areas like finance and customer service.
Performance, Testing & Cost Optimization
Even the best-designed agents need monitoring, reinforcement learning, and testing.
Salesforce has built an internal Testing Center that allows simulation of multi-turn conversations, injection attack tests, and gap identification. This ensures agents don’t hallucinate or leak sensitive data.
Performance metrics include;
- Accuracy of response
- Deflection rates
- Off-topic interaction rates
- Latency (<5 seconds preferred)
A single contact center interaction costs ~$10 on average. If AI agents bring that down to $2, companies could save millions annually.
What’s Next: Agent Exchange and Expansion
Salesforce is building toward an “AppExchange for Agents” – a marketplace where industry-specific agents can be created, customized, and deployed, just like apps.
Use cases include;
- Agents for ERP platforms
- Industry-specific verticals (retail, BFSI, healthcare)
- Partner-built agents sold via ecosystem
Expect growing partner-led use cases as part of the Digital Labor Platform narrative Marc Benioff has endorsed.
Current Challenges: Adoption and Cost Clarity
Despite excitement, real-world deployment faces two hurdles;
- Production-Ready Agents – Many companies build agents but delay production due to testing gaps.
- Complex Pricing – Especially when combining Agentforce with Data Cloud credits.
Salesforce is working to simplify pricing through Data Cloud Wallet and clearer matrices. But as Manjeet puts it;
“If the value is high enough, pricing will catch up. The solution has to solve real problems first.”
The Fitness of AI (and Vice Versa)
The episode ends with a powerful analogy – Training an AI model is like training your body.
- Overfitting = doing only yoga without strength training
- Feedback loops = measuring progress and adapting
- Meta skills = quality sleep and consistent workouts in fitness; good prompting and evaluation in AI
“How you ask questions to AI is the new literacy,” Manjeet says – a line every tech leader should remember.
Final Thought
Agentforce isn’t just a Salesforce product. It represents the shift from static systems to autonomous workflows, redefining productivity in CRM and beyond.
If you’re a business leader evaluating where to place your AI bets, Agentforce provides not just tools – but a strategic foundation for future-ready operations.
🎧 Listen to the full episode on YouTube
Are you ready to break down the silos and build a connected future?
Let’s start with a quick strategy session to map your interoperability journey.