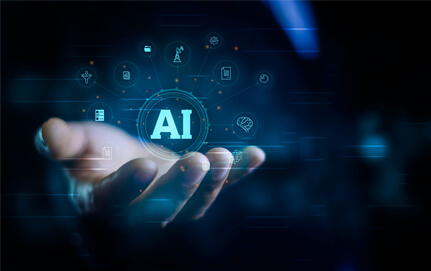
The Enterprise AI Playbook – Insights and Strategies from Real-World Enterprise AI Implementations
By Teqfocus Team
9th July, 2024
Table of contents
Introduction
The State of Enterprise AI Adoption
Emerging AI Technologies and Their Applications
AI Strategy and Leadership
Data Infrastructure and AI Readiness
AI Implementation Best Practices
Overcoming AI Implementation Challenges
Industry-Specific AI Applications and Case Studies
The Future of Enterprise AI
Conclusion
Artificial Intelligence (AI) has moved beyond buzzword status to become a critical driver of business transformation across industries. As organizations navigate the complex landscape of AI adoption, understanding real-world implementation strategies and outcomes has never been more crucial.
In this comprehensive analysis, we dive deep into the insights gleaned from over 500+ conversations with enterprise leaders, AI practitioners, and industry experts. Our goal is to provide a clear, actionable roadmap for organizations looking to harness the power of AI to drive innovation, efficiency, and competitive advantage.
From machine learning algorithms optimizing supply chains to natural language processing revolutionizing customer service, we’ll explore how AI is reshaping the enterprise landscape. We’ll uncover the challenges faced, strategies employed, and lessons learned by companies at various stages of their AI journey.
The State of Enterprise AI Adoption – A Deep Dive into Generative AI
As we navigate the rapidly evolving landscape of artificial intelligence in the enterprise, our extensive conversations with industry leaders have revealed a significant shift towards generative AI. This powerful technology is reshaping how businesses operate, innovate, and compete in today’s data-driven world.
Current Adoption Rates and Future Projections
The adoption of generative AI in enterprise settings is accelerating at an unprecedented pace. While exact figures vary by industry, our research indicates that a majority of large enterprises are either actively implementing or seriously considering generative AI solutions.
According to a recent survey by McKinsey, 50% of respondents report that their organizations have adopted AI in at least one business function, with generative AI being a key focus area for many.
Looking ahead, Gartner predicts that by 2025, 30% of outbound marketing messages from large organizations will be synthetically generated, highlighting the growing importance of generative AI in business operations.
Key Areas of Generative AI Implementation
Our research has identified four critical areas where generative AI is making significant impacts in enterprise settings;
1. Data Integration
Current Utilization
- Integration with Existing Data Sources: Companies are actively integrating generative AI with their existing data infrastructure through APIs and data lakes. This integration allows for seamless access and analysis of both structured and unstructured data.
- Digitizing Unstructured Data: On average, enterprises report that approximately 60% of their data is structured, while 40% is unstructured. AI tools are being employed to automate the digitization of unstructured data such as emails, reports, and multimedia, converting them into structured formats that are easier to analyze.
Planned Utilization
- Expanding AI Capabilities: Enterprises are planning to enhance their AI systems to handle more complex data types, including real-time streaming data. This expansion aims to improve decision-making processes and operational efficiency.
- Focus on Unstructured Data: Future projects will emphasize the digitization and integration of unstructured data sources, such as social media content and customer feedback, to gain deeper insights.
- Incorporating New Data Sources: Companies intend to integrate data from IoT devices and external market sources to enrich their AI models and enhance predictive accuracy.
2. Relevancy of Insights
Current Utilization
- Industry-Specific Insights: Generative AI is being tailored to provide industry-specific insights by training models on relevant datasets. This ensures that the outputs are pertinent to each business’s unique context.
- Integration into Business Processes: AI-generated insights are being incorporated into key business processes such as supply chain management, customer relationship management, and financial forecasting.
- Measuring Relevance and Accuracy: Companies are utilizing performance metrics, user feedback, and continuous model evaluation to assess the relevancy and accuracy of AI-generated insights.
Planned Utilization
- Improving Relevancy: Future initiatives include refining AI models with more granular industry data and incorporating advanced machine learning techniques to improve the precision of insights.
- Enhancing Business Processes: Plans are in place to extend AI integration into additional business processes, such as human resources and compliance, to further streamline operations.
- Precision Goals: Enterprises aim to achieve higher precision in AI insights by leveraging more sophisticated algorithms and expanding their data inputs to cover broader industry trends.
3. User Adoption
Current Utilization
- Frequency of AI Tool Usage: End-users in enterprises are utilizing generative AI tools on a daily basis, primarily for data analysis, report generation, and predictive analytics.
- User Feedback: While AI tools are generally perceived as helpful, feedback indicates room for improvement in user interface design and tool intuitiveness.
- Adoption Support: Companies are supporting user adoption through comprehensive training programs, dedicated support teams, and regular updates to AI tools based on user feedback.
Planned Utilization
- Increasing Adoption: Strategies to boost user adoption include developing more user-friendly interfaces, offering personalized AI features, and enhancing training programs.
- New AI Tool Introduction: Enterprises plan to roll out new AI tools that offer greater customization and are tailored to specific user roles, ensuring they meet diverse needs.
- Success Measurement: The success of AI adoption will be measured through user satisfaction surveys, usage metrics, and the impact of AI on productivity and decision-making.
4. Infrastructure
Current Utilization
- Security Measures: Advanced encryption, access controls, and regular security audits are being implemented to protect AI systems from breaches.
- Scalability Solutions: Scalable infrastructure is achieved through cloud-based solutions, containerization, and microservices architecture, enabling easy scaling of AI applications.
- Governance and Compliance: Governance frameworks are in place to ensure compliance with industry regulations and standards, including data privacy laws and ethical AI guidelines.
Planned Utilization
- Enhanced Security: Future plans involve adopting zero-trust security models and incorporating AI-driven security analytics to proactively identify and mitigate threats.
- Infrastructure Scaling: Companies plan to invest in more robust cloud infrastructure and edge computing solutions to support the growing demands of AI applications.
- Governance Policies: New governance policies will focus on transparent AI practices, bias mitigation, and regular audits to ensure ethical and compliant use of AI technologies.
Challenges and Opportunities
While the adoption of generative AI presents immense opportunities, enterprises face several challenges;
- Data Quality and Integration: Ensuring high-quality, diverse data sets for training AI models remains a significant hurdle.
- Skill Gaps: The shortage of AI talent continues to be a bottleneck for many organizations.
- Ethical Considerations: Navigating the ethical implications of AI, particularly in areas like bias and privacy, is an ongoing concern.
- ROI Measurement: Quantifying the return on investment for AI initiatives can be complex, making it challenging to justify large-scale implementations.
Despite these challenges, the potential benefits of generative AI – from increased operational efficiency to enhanced decision-making capabilities – are driving continued investment and innovation in this space.
As we move forward in this blog post, we’ll explore these trends in greater depth, examining the specific technologies driving AI adoption, the strategies employed by successful implementers, and the future outlook for generative AI in the enterprise landscape.
Emerging AI Technologies and Their Enterprise Applications
As generative AI continues to evolve, it’s reshaping the landscape of enterprise technologies. Our research has revealed several key areas where AI is making significant impacts, driving innovation and efficiency across various industries.
Machine Learning and Deep Learning
Machine Learning (ML) and Deep Learning (DL) form the foundation of many generative AI applications, enabling systems to learn from data and improve their performance over time.
Current Applications
- Predictive Analytics: Enterprises are leveraging ML algorithms to forecast trends, from sales projections to equipment maintenance needs. For instance, a study by Salesforce found that 33% of marketers are using AI for predictive analytics in their campaigns.
- Natural Language Generation: ML-powered systems are being used to generate human-like text for various applications, from automated reporting to personalized customer communications.
- Image and Video Generation: Deep learning models like GANs (Generative Adversarial Networks) are enabling the creation of realistic images and videos, with applications in product design, marketing, and entertainment.
Future Trends
- Few-Shot Learning: This emerging technique allows AI models to learn from smaller datasets, making them more adaptable and efficient.
- Explainable AI: As AI systems become more complex, there’s a growing focus on developing models that can explain their decision-making processes, crucial for building trust and meeting regulatory requirements.
Natural Language Processing and Conversational AI
Natural Language Processing (NLP) is revolutionizing how enterprises interact with textual data and communicate with customers.
Current Applications
- Intelligent Chatbots: Advanced NLP is powering more sophisticated chatbots, capable of handling complex customer queries. According to a report by MIT Technology Review, businesses using AI chatbots have seen customer service costs reduced by up to 30%.
- Sentiment Analysis: Companies are using NLP to analyze customer feedback across various channels, gaining insights into brand perception and product satisfaction.
- Document Processing: NLP is being employed to extract key information from vast document repositories, streamlining processes in legal, finance, and healthcare sectors.
Future Trends
- Multilingual NLP: As businesses become increasingly global, there’s a growing demand for NLP systems that can operate seamlessly across multiple languages.
- Emotion AI: The next frontier in NLP involves understanding and responding to human emotions, opening up new possibilities in areas like mental health support and personalized education.
Computer Vision
Computer vision technologies are transforming how businesses interact with visual data, from quality control in manufacturing to medical imaging in healthcare.
Current Applications
- Quality Assurance: Manufacturers are using computer vision systems to detect defects in products at speeds and accuracies impossible for human inspectors. For example, BMW has implemented AI-powered visual inspection systems that have reduced quality control errors by up to 30%.
- Retail Analytics: Retailers are employing computer vision to analyze in-store customer behavior, optimize product placement, and even enable cashier-less stores.
- Medical Imaging: Healthcare providers are leveraging AI-powered image analysis to assist in diagnosing diseases from X-rays, MRIs, and other medical imaging techniques.
Future Trends
- 3D Vision: Advancements in 3D computer vision are opening up new possibilities in areas like augmented reality and robotics.
- Edge AI: The integration of computer vision capabilities into edge devices is set to enable real-time analysis in areas like autonomous vehicles and smart cities.
Robotic Process Automation (RPA) and AI
The combination of RPA and AI is revolutionizing how businesses handle repetitive tasks and workflows.
Current Applications
- Finance and Accounting: Companies are using AI-enhanced RPA to automate invoice processing, reconciliations, and financial reporting. Gartner predicts that by 2025, organizations will lower operational costs by 30% by combining hyperautomation technologies with redesigned operational processes.
- Human Resources: HR departments are leveraging RPA and AI for resume screening, employee onboarding, and benefits management.
- Supply Chain Management: Logistics companies are employing AI-powered RPA to optimize routing, inventory management, and demand forecasting.
Future Trends
- Cognitive Automation: The next wave of RPA will incorporate more advanced AI capabilities, enabling the automation of complex, judgment-based tasks.
- Self-Learning RPA: Future RPA systems will be able to learn from their interactions and continuously improve their performance without human intervention.
Data Integration and AI
As highlighted in our research, data integration is a critical focus area for enterprises leveraging AI.
Current Applications
- Data Lakes and Warehouses: Companies are building comprehensive data repositories that combine structured and unstructured data, providing a rich foundation for AI models.
- Real-time Data Processing: Enterprises are implementing stream processing technologies to handle real-time data, enabling more responsive AI applications.
Future Trends
- Edge Computing: The rise of edge computing will allow for more efficient data processing and AI model deployment closer to the data source.
- Federated Learning: This emerging technique allows AI models to be trained across multiple decentralized devices or servers holding local data samples, addressing privacy concerns and enabling more collaborative AI development.
As these technologies continue to evolve, their impact on enterprise operations is set to grow exponentially. In the next section, we’ll explore how organizations are developing strategies to leverage these technologies effectively and the role of leadership in driving AI initiatives.
AI Strategy and Leadership – Driving Enterprise Transformation
As generative AI continues to revolutionize business operations, effective strategy and leadership have become crucial for successful implementation and adoption. Our research has uncovered key insights into how forward-thinking enterprises are approaching AI strategy and the pivotal role of leadership in driving AI initiatives.
Building an AI-Ready Organization
Creating an AI-ready organization involves more than just implementing technology; it requires a fundamental shift in organizational culture and mindset.
Current Approaches
- Data-Driven Culture: Leading organizations are fostering a data-driven culture where decisions at all levels are informed by insights derived from AI and analytics. According to a study by NewVantage Partners, 92.2% of leading companies report that they are increasing their investments in data and AI initiatives.
- Cross-Functional Collaboration: Successful AI implementations often require collaboration across different departments. Companies are creating cross-functional teams that bring together domain experts, data scientists, and IT professionals to drive AI projects.
- Continuous Learning: Organizations are investing in ongoing training and education programs to keep their workforce up-to-date with the latest AI developments. A report by Deloitte found that 68% of executives say that their workers will need to learn new skills to do their jobs in the next three years due to AI and automation.
Future Trends
- AI Literacy Programs: Companies are planning to implement organization-wide AI literacy programs to ensure all employees have a basic understanding of AI capabilities and limitations.
- Ethical AI Frameworks: As AI becomes more pervasive, organizations are developing comprehensive ethical AI frameworks to guide decision-making and ensure responsible AI use.
The Role of the C-Suite in Driving AI Initiatives
Executive leadership plays a critical role in the success of AI initiatives. Our conversations with industry leaders have highlighted several key areas where C-suite involvement is crucial.
Current Practices
- Setting the AI Vision: CEOs and other top executives are actively involved in setting the overall AI vision for their organizations, aligning it with broader business strategies.
- Resource Allocation: C-suite leaders are making strategic decisions about resource allocation for AI initiatives, balancing short-term gains with long-term transformation goals.
- Risk Management: With the increasing importance of AI, executives are taking a more active role in understanding and managing AI-related risks, including data privacy, security, and ethical concerns.
Future Trends
- AI-Savvy Boards: There’s a growing trend towards appointing board members with AI expertise to provide better oversight and guidance on AI strategies.
- Chief AI Officer (CAIO): Some organizations are creating new C-level positions, such as Chief AI Officer, to lead and coordinate AI efforts across the enterprise.
Developing AI Talent and Fostering a Data-Driven Culture
Addressing the AI skills gap and cultivating a data-driven culture are critical challenges for many organizations.
Current Strategies
- Talent Acquisition and Retention: Companies are implementing aggressive hiring strategies to attract top AI talent. According to a report by LinkedIn, AI specialists are among the top emerging jobs, with 74% annual growth in job postings.
- Upskilling and Reskilling: Organizations are investing heavily in upskilling their existing workforce. Amazon, for instance, has pledged $700 million to retrain 100,000 employees in technology skills, including AI and machine learning.
- Partnerships with Academia: Many enterprises are forming partnerships with universities to nurture AI talent and stay at the forefront of AI research.
Future Trends
- AI Residency Programs: Following the lead of tech giants, more companies are planning to implement AI residency programs to attract and develop top AI talent.
- Democratizing AI Development: There’s a growing focus on developing tools and platforms that allow non-technical employees to create and deploy AI models, democratizing AI development across the organization.
Measuring ROI and Demonstrating Value
Quantifying the return on investment (ROI) for AI initiatives remains a challenge for many organizations, but it’s crucial for securing ongoing support and funding.
Current Approaches
- KPI Alignment: Companies are aligning AI project KPIs with broader business objectives to demonstrate tangible value. For example, a chatbot implementation might be measured not just by customer satisfaction scores, but also by reduction in call center costs.
- Pilot Projects: Organizations are using pilot projects to demonstrate AI value quickly and build support for larger initiatives. A study by Boston Consulting Group found that companies that scale up from pilots to multiple use cases achieve significantly higher ROI.
Future Trends
- AI Value Dashboards: Companies are developing comprehensive dashboards that track the value generated by AI across various business functions, providing real-time visibility into AI ROI.
- Long-Term Value Metrics: There’s a growing recognition of the need to develop metrics that capture the long-term transformational value of AI, beyond immediate cost savings or revenue increases.
As we’ve seen, successful AI implementation requires more than just technological prowess; it demands strategic vision, strong leadership, and a commitment to fostering a data-driven, AI-ready culture.
Data Infrastructure and AI Readiness – Building the Foundation for Success
The effectiveness of AI initiatives in the enterprise is fundamentally tied to the quality and accessibility of data. Our research has shown that organizations with robust data infrastructure are better positioned to leverage AI for significant business impact. This section explores the critical components of data infrastructure and AI readiness.
The Importance of a Robust Data Foundation
A solid data foundation is the bedrock upon which successful AI initiatives are built. It encompasses data collection, storage, processing, and governance.
Current Practices
- Data Lakes and Data Warehouses: Enterprises are increasingly adopting modern data architectures that combine the flexibility of data lakes with the structure of data warehouses. According to a survey by Deloitte, 64% of companies are investing in data lakes to improve their analytics and AI capabilities.
- Real-time Data Processing: Organizations are implementing stream processing technologies to handle real-time data, enabling more responsive AI applications. For instance, retail giants like Walmart are using real-time data processing to optimize inventory and pricing decisions.
- Data Quality Initiatives: Companies are investing heavily in data quality tools and processes. A study by Gartner found that poor data quality costs organizations an average of $12.9 million annually.
Future Trends
- Data Fabric Architecture: This emerging concept aims to create a unified data management framework that works across disparate data sources and platforms, simplifying data integration for AI applications.
- DataOps: Similar to DevOps in software development, DataOps practices are being adopted to improve the speed and reliability of data analytics pipelines.
Cloud Computing and AI – A Symbiotic Relationship
Cloud computing has emerged as a critical enabler for AI initiatives, providing the scalable compute power and storage necessary for complex AI workloads.
Current Utilization
- Hybrid and Multi-cloud Strategies: Organizations are adopting hybrid and multi-cloud approaches to balance flexibility, performance, and cost. According to a report by, 93% of enterprises have a multi-cloud strategy.
- AI-as-a-Service (AIaaS): Companies are leveraging cloud-based AI services to quickly deploy AI capabilities without significant upfront investment. Major cloud providers like AWS, Google Cloud, and Microsoft Azure offer a range of AIaaS solutions.
- GPU-accelerated Computing: Cloud providers are offering GPU-accelerated computing instances, which are particularly well-suited for training deep learning models.
Future Trends
- Edge-Cloud Continuum: As edge computing grows, we’re seeing a trend towards seamless integration between edge devices and cloud infrastructure, enabling more efficient AI model deployment and data processing.
- Quantum Computing: While still in its early stages, quantum computing holds promise for dramatically accelerating certain types of AI computations. Major tech companies and startups are investing heavily in this area.
Data Governance and Ethics in the Age of AI
As AI becomes more pervasive, robust data governance and ethical considerations are becoming increasingly critical.
Current Approaches
- Data Privacy Compliance: Organizations are implementing comprehensive data governance frameworks to ensure compliance with regulations like GDPR and CCPA. A survey by KPMG found that 75% of organizations consider data protection and privacy as the top challenge in AI adoption.
- Ethical AI Frameworks: Companies are developing ethical AI guidelines to ensure responsible AI use. For example, Microsoft has published AI ethics principles that guide their AI development and deployment.
- Bias Detection and Mitigation: Organizations are implementing tools and processes to detect and mitigate bias in AI models. IBM’s AI Fairness 360 toolkit is an example of an open-source solution in this space.
Future Trends
- Explainable AI: There’s a growing focus on developing AI models that can explain their decision-making processes, crucial for building trust and meeting regulatory requirements.
- AI Auditing: We anticipate the rise of AI auditing practices, both internal and external, to ensure AI systems are behaving as intended and adhering to ethical guidelines.
Scalability and Performance Optimization
As AI applications grow in complexity and scale, optimizing performance becomes increasingly important.
Current Practices
- Distributed Computing: Organizations are leveraging distributed computing frameworks like Apache Spark to process large datasets efficiently.
- Model Optimization: Techniques like model compression and quantization are being used to deploy AI models on resource-constrained devices.
Future Trends
- AutoML for Infrastructure: We’re seeing the emergence of AutoML tools that not only automate model selection but also optimize infrastructure choices for AI workloads.
- Neuromorphic Computing: This emerging field aims to create AI hardware that mimics the structure and function of biological neural networks, potentially offering significant performance and energy efficiency improvements.
Building a robust data infrastructure and ensuring AI readiness is a complex but crucial task. Organizations that get this right are well-positioned to reap significant benefits from their AI initiatives.
AI Implementation Best Practices – Lessons from the Frontlines
As enterprises continue to navigate the complex landscape of AI adoption, certain best practices have emerged from successful implementations. Our research and conversations with industry leaders have revealed key strategies that can significantly improve the chances of AI project success.
Starting Small – The Power of Pilot Projects
One of the most consistent recommendations from successful AI implementers is to start with small, focused pilot projects.
Current Approaches
- Proof of Concept (POC) Projects: Organizations are using POC projects to test AI technologies in a controlled environment before wider deployment. According to a survey by Gartner, 75% of organizations that have successfully scaled AI began with proof of concept projects.
- Agile Methodology: Companies are adopting agile methodologies for AI projects, allowing for rapid iteration and learning. This approach helps in quickly identifying what works and what doesn’t.
- Clear Success Metrics: Successful organizations define clear, measurable success criteria for their pilot projects. These metrics are often tied to specific business outcomes rather than technical performance alone.
Best Practice Example: Procter & Gamble (P&G) successfully used a pilot project approach to implement AI in their supply chain. They started with a small-scale project to optimize inventory levels in a single product category. The success of this pilot led to a broader rollout across their supply chain, resulting in significant cost savings and improved efficiency.
Scaling AI Across the Enterprise
Once pilot projects prove successful, the next challenge is scaling AI across the organization.
Current Strategies
- Center of Excellence (CoE) Model: Many organizations are establishing AI Centers of Excellence to centralize expertise and coordinate AI efforts across different business units. A study by Deloitte found that companies with a central AI CoE are 1.5 times more likely to be successful in scaling AI.
- Standardization and Reusability: Successful organizations focus on creating reusable AI components and standardized processes to accelerate deployment across different use cases.
- Change Management: Companies are recognizing the importance of robust change management processes in AI implementation. This includes communication strategies, training programs, and efforts to address employee concerns about AI adoption.
Best Practice Example: JPMorgan Chase has successfully scaled AI across its operations by establishing a central AI team and developing a common AI platform called OmniAI. This platform allows different business units to leverage AI capabilities consistently, accelerating adoption across the organization.
Measuring ROI and Demonstrating Value
Quantifying the return on investment (ROI) for AI initiatives remains a challenge for many organizations, but it’s crucial for securing ongoing support and funding.
Current Approaches
- Business-Aligned KPIs: Leading organizations are aligning AI project KPIs with broader business objectives. For example, an AI-powered chatbot might be measured not just by technical performance, but by reduction in call center costs and improvement in customer satisfaction scores.
- Total Value of Ownership (TVO): Companies are moving beyond traditional ROI calculations to consider the Total Value of Ownership, which includes factors like improved decision-making capabilities and increased innovation potential.
- Continuous Monitoring: Successful implementers are setting up systems for continuous monitoring and reporting of AI project performance, allowing for real-time adjustments and optimization.
Best Practice Example: American Express has implemented a comprehensive AI value tracking system. They measure the impact of their AI initiatives not just in terms of cost savings, but also in improved customer experiences and fraud detection capabilities. This holistic approach has helped them justify continued investment in AI technologies.
Ensuring Ethical AI Implementation
As AI becomes more pervasive, ensuring ethical implementation has become a critical best practice.
Current Strategies
- Ethical AI Frameworks: Organizations are developing comprehensive ethical AI guidelines to ensure responsible AI use. For instance, IBM has published its Everyday Ethics for AI, providing practical guidance for AI developers and users.
- Diverse AI Teams: Companies are focusing on building diverse AI teams to help mitigate bias in AI systems. Research has shown that diverse teams are better at identifying and addressing potential ethical issues in AI.
- Transparency and Explainability: Leading organizations are prioritizing the development of explainable AI models, particularly in high-stakes applications like healthcare and finance.
Best Practice Example: Microsoft has taken a leading role in ethical AI implementation. They’ve established an AI ethics review process that all AI projects must go through before deployment. This process involves a multidisciplinary team that assesses potential ethical risks and ensures alignment with Microsoft’s AI principles.
Fostering a Culture of Continuous Learning
Given the rapid pace of AI advancement, fostering a culture of continuous learning is crucial for long-term success.
Current Approaches
- Internal AI Training Programs: Companies are investing in comprehensive AI training programs for employees across different roles. For example, Amazon’s Machine Learning University, initially created for internal use, is now available to the public.
- Partnerships with Academia: Organizations are forming partnerships with universities to stay at the forefront of AI research and to nurture AI talent.
- AI Communities of Practice: Leading companies are establishing internal AI communities of practice to facilitate knowledge sharing and collaboration across different teams and departments.
Best Practice Example: Google has implemented a successful continuous learning approach through its “AI Residency Program.” This program brings in top talent for a year-long residency, allowing them to work on cutting-edge AI projects while learning from Google’s AI experts.
By following these best practices, organizations can significantly improve their chances of successful AI implementation.
Overcoming AI Implementation Challenges – Navigating the Pitfalls
While the potential benefits of AI are substantial, organizations often encounter significant challenges during implementation. Our research has identified several common hurdles and effective strategies to address them.
Addressing Data Quality and Availability Issues
Data is the lifeblood of AI systems, and poor data quality or availability can derail even the most promising AI initiatives.
Challenges
- Data Silos: Many organizations struggle with data trapped in disparate systems, making it difficult to create a comprehensive view for AI analysis.
- Inconsistent Data Formats: Varying data formats across different systems can complicate data integration efforts.
- Data Privacy Concerns: Stricter data privacy regulations like GDPR and CCPA can limit data availability for AI training.
Solutions
- Data Unification Platforms: Companies are investing in data unification platforms to break down silos and create a single source of truth for AI applications.
- Data Governance Programs: Implementing robust data governance programs helps ensure data quality and consistency across the organization.
- Synthetic Data Generation: To address privacy concerns and data scarcity, organizations are exploring synthetic data generation techniques. A study by Gartner predicts that by 2025, 60% of the data used for AI and analytics projects will be synthetically generated.
Best Practice Example: Airbnb faced challenges with inconsistent data across its global operations. They implemented a comprehensive data quality initiative, including a central data catalog and automated data quality checks. This effort significantly improved their ability to leverage AI for personalized recommendations and pricing optimization.
Managing Change and Fostering User Adoption
Resistance to change and low user adoption can significantly hinder AI implementation efforts.
Challenges
- Fear of Job Displacement: Employees may resist AI adoption due to concerns about job security.
- Lack of AI Literacy: Many employees may not understand AI capabilities and limitations, leading to unrealistic expectations or underutilization.
- Inadequate Training: Insufficient training can result in poor user adoption of AI tools.
Solutions:
- Clear Communication: Organizations are developing comprehensive communication strategies to explain the role of AI and its impact on jobs, emphasizing augmentation rather than replacement.
- AI Literacy Programs: Companies are implementing organization-wide AI literacy programs to ensure all employees have a basic understanding of AI.
- Hands-on Training: Successful implementers are providing hands-on, role-specific training for AI tools to improve adoption rates.
Best Practice Example: Walmart has successfully managed the change associated with AI implementation through its Walmart Academy program. This program provides employees with training on new technologies, including AI, helping them understand how these tools can enhance their work rather than replace them.
Ethical and Regulatory Considerations
As AI becomes more pervasive, organizations must grapple with complex ethical and regulatory challenges.
Challenges
- Algorithmic Bias: AI systems can perpetuate or amplify existing biases, leading to unfair outcomes.
- Lack of Explainability: Many AI models, particularly deep learning models, can be difficult to interpret, creating challenges in regulated industries.
- Rapidly Evolving Regulations: The regulatory landscape for AI is constantly changing, making compliance a moving target.
Solutions
- Ethical AI Frameworks: Organizations are developing comprehensive ethical AI guidelines to ensure responsible AI use.
- Explainable AI Techniques: Companies are investing in techniques to make AI models more interpretable and explainable.
- Cross-functional AI Governance: Leading organizations are establishing cross-functional AI governance teams to address ethical and regulatory challenges.
Best Practice Example: IBM has taken a proactive approach to ethical AI with its AI Ethics Board and the development of AI Fairness 360, an open-source toolkit to help detect and mitigate bias in AI models. This approach has helped IBM navigate the complex ethical landscape of AI implementation.
Talent Acquisition and Retention
The shortage of AI talent remains a significant challenge for many organizations.
Challenges
- Competitive Job Market: Demand for AI specialists far outstrips supply, leading to intense competition for talent.
- Rapid Skill Obsolescence: The fast-paced nature of AI development means skills can quickly become outdated.
- Retention Challenges: AI specialists are often attracted to cutting-edge projects, making it difficult for non-tech companies to retain talent.
Solutions
- Upskilling Programs: Organizations are investing heavily in upskilling their existing workforce.
- Partnerships with Academia: Companies are forming partnerships with universities to nurture AI talent and stay at the forefront of AI research.
- Creating Attractive Work Environments: Organizations are focusing on creating work environments that appeal to AI talent, including opportunities to work on cutting-edge projects and contribute to open-source initiatives.
Technical Challenges in AI Implementation
Organizations often face technical hurdles in deploying and scaling AI solutions.
Challenges
- Integration with Legacy Systems: Many organizations struggle to integrate AI solutions with existing legacy IT infrastructure.
- Scalability Issues: AI models that work well in small-scale pilots may face challenges when scaled to production environments.
- Model Drift: AI models can degrade over time as the underlying data distributions change, a phenomenon known as model drift.
Solutions:
- Microservices Architecture: Companies are adopting microservices architectures to make it easier to integrate AI capabilities with existing systems.
- Cloud-based AI Platforms: Organizations are leveraging cloud-based AI platforms to address scalability challenges.
- Continuous Monitoring and Retraining: Leading companies are implementing systems for continuous monitoring of AI model performance and automated retraining to address model drift.
Best Practice Example: Netflix has successfully addressed many technical challenges in AI implementation through its microservices architecture and advanced monitoring systems. Their recommendation system, which relies heavily on AI, is built as a collection of microservices that can be easily scaled and updated. They also employ sophisticated monitoring tools to detect and address issues like model drift in real-time.
By understanding these common challenges and learning from successful implementations, organizations can significantly improve their chances of AI project success.
AI’s Impact on the Workforce – Navigating the Changing Landscape of Work
As AI continues to reshape industries, its impact on the workforce has become a topic of intense discussion and concern. Our research has revealed a nuanced picture of AI’s effects on employment, skill requirements, and the nature of work itself.
Job Displacement vs. Job Creation
One of the most pressing concerns about AI is its potential to automate jobs, leading to widespread unemployment. However, the reality is more complex.
Current Trends
- Job Displacement: Certain roles, particularly those involving routine, repetitive tasks, are indeed at risk of automation. A study by McKinsey Global Institute estimates that by 2030, up to 375 million workers (14% of the global workforce) may need to switch occupational categories due to AI and automation.
- Job Creation: At the same time, AI is creating new job categories and increasing demand for certain skills. The World Economic Forum predicts that while 75 million jobs may be displaced by AI, 133 million new jobs may emerge as a result of AI adoption.
- Job Transformation: Many existing roles are being transformed rather than eliminated, with AI augmenting human capabilities rather than replacing them entirely.
Best Practice Example: JPMorgan Chase has taken a proactive approach to job transformation in the face of AI adoption. They’ve implemented a program called “Skills Passport” that helps employees identify how their roles might change due to AI and provides training for new, in-demand skills. This approach has helped the company redeploy talent affected by automation to new roles within the organization.
Upskilling and Reskilling for the AI Era
As AI transforms the job market, there’s an urgent need for widespread upskilling and reskilling initiatives.
Current Approaches
- Corporate Training Programs: Many companies are investing heavily in employee training programs. For instance, PwC has pledged $3 billion to upskill all of its 275,000 employees over the next few years, with a focus on digital and AI skills.
- Partnerships with Educational Institutions: Organizations are partnering with universities and online education platforms to provide employees with access to AI and data science courses.
- Apprenticeship Programs: Some companies are implementing apprenticeship programs to train employees in AI-related roles, combining on-the-job training with formal education.
Future Trends
- Lifelong Learning: There’s a growing recognition that continuous learning will be essential in the AI era. We expect to see more companies adopting “learning sabbatical” policies and providing ongoing education benefits.
- Personalized Learning Paths: AI itself is being used to create personalized learning experiences, tailoring training programs to individual employee needs and learning styles.
Best Practice Example: AT&T’s Future Ready program is a prime example of a comprehensive upskilling initiative. The company invested $1 billion in a multi-year program to retrain nearly half of its workforce for new, technology-oriented roles. The program includes online courses, nanodegrees, and partnerships with universities.
Human-AI Collaboration – The Future of Work
Rather than a wholesale replacement of human workers, the future of work is increasingly seen as one of human-AI collaboration.
Current Trends
- AI as a Co-worker: In many fields, AI is being deployed as a tool to augment human capabilities rather than replace them entirely. For example, in healthcare, AI is assisting doctors with diagnosis and treatment planning, but not replacing the need for human medical professionals.
- New Human-AI Interfaces: Organizations are developing new interfaces that allow for more intuitive interaction between humans and AI systems. This includes natural language interfaces and augmented reality displays that overlay AI insights onto the physical world.
- AI-Enabled Decision Making: AI is increasingly being used to provide insights and recommendations to human decision-makers, rather than making decisions autonomously.
Future Trends
- AI Coaches and Mentors: We anticipate the development of AI systems that can serve as personal coaches or mentors, helping employees improve their skills and performance over time.
- Cognitive Prosthetics: Advanced AI systems may serve as “cognitive prosthetics,” enhancing human cognitive abilities in areas like memory recall and complex problem-solving.
Best Practice Example: Stitch Fix, an online personal styling service, provides an excellent example of effective human-AI collaboration. Their business model relies on a combination of AI algorithms and human stylists. The AI system analyzes customer data and preferences to make initial recommendations, but human stylists make the final selections, adding a personal touch that AI alone cannot provide.
Addressing the Psychological Impact of AI in the Workplace
As AI becomes more prevalent in the workplace, organizations need to address its psychological impact on employees.
Current Approaches
- Transparent Communication: Leading companies are prioritizing clear communication about AI initiatives, including their purpose, limitations, and impact on jobs.
- Empowerment Through AI: Organizations are framing AI as a tool for employee empowerment, helping workers focus on more meaningful and creative aspects of their jobs.
- Mental Health Support: Some companies are expanding their mental health support services to help employees cope with the stress and uncertainty associated with technological change.
Future Trends
- AI Ethics Training: We expect to see more organizations implementing AI ethics training for employees, helping them understand and navigate the ethical implications of AI in their work.
- Human-Centered AI Design: There’s a growing focus on designing AI systems with human psychological needs in mind, aiming to create AI tools that are not just efficient but also satisfying and meaningful for humans to work with.
Best Practice Example: Microsoft has taken a leading role in addressing the psychological impact of AI in the workplace. They’ve developed guidelines for human-AI interaction that prioritize transparency, fairness, and user control. These guidelines inform the design of their AI products and services, aiming to create AI systems that empower rather than alienate human workers.
As AI continues to transform the workplace, organizations that proactively address these challenges and opportunities will be best positioned to thrive.
Industry-Specific AI Applications and Case Studies – AI in Action
While AI has broad applications across industries, its implementation and impact can vary significantly depending on the sector. In this section, we’ll explore how different industries are leveraging AI to drive innovation, improve efficiency, and create new value propositions.
Finance and Banking
The finance and banking sector has been at the forefront of AI adoption, using the technology to enhance customer experience, improve risk management, and streamline operations.
Key Applications
- Fraud Detection: AI algorithms can analyze vast amounts of transaction data in real-time to identify potentially fraudulent activities.
- Algorithmic Trading: AI-powered trading systems can make high-speed trading decisions based on market data analysis.
- Personalized Banking: AI is being used to provide personalized financial advice and product recommendations to customers.
Case Study: Teqfocus implemented an AI system called TeqIDP to analyze commercial loan agreements. The system can review documents in seconds that would take lawyers and loan officers 360,000 hours annually. This has not only improved efficiency but also reduced loan-servicing mistakes.
Future Trends
- AI-Powered Risk Assessment: Sophisticated AI models for credit risk assessment, potentially opening up new lending opportunities.
- Autonomous Finance: The concept of “self-driving finance,” where AI systems manage individuals’ finances with minimal human intervention, is gaining traction.
Healthcare and Life Sciences
AI is transforming healthcare, from drug discovery to patient care and hospital management.
Key Applications
- Diagnostic Assistance: AI algorithms can analyze medical images to assist in the diagnosis of diseases like cancer.
- Drug Discovery: AI is accelerating the drug discovery process by predicting how different chemical compounds will behave.
- Personalized Treatment Plans: AI can analyze patient data to recommend personalized treatment plans.
Case Study: Google DeepMind and NHS Collaboration Google DeepMind collaborated with the UK’s National Health Service to develop an AI system that can analyze eye scans and detect over 50 eye diseases with accuracy comparable to expert doctors. This system has the potential to expedite diagnosis and treatment, particularly in areas with a shortage of specialists.
Future Trends
- AI in Genomics: Increased use of AI in analyzing genomic data for personalized medicine.
- Robot-Assisted Surgery: AI-powered surgical robots are likely to become more sophisticated and widely adopted.
Manufacturing and Supply Chain
AI is revolutionizing manufacturing and supply chain management, improving efficiency, quality control, and predictive maintenance.
Key Applications
- Predictive Maintenance: AI can predict when equipment is likely to fail, allowing for proactive maintenance.
- Quality Control: Computer vision systems can detect defects in products at speeds and accuracies impossible for human inspectors.
- Supply Chain Optimization: AI can analyze vast amounts of data to optimize inventory levels and logistics.
Case Study: Siemens’ AI-Powered Gas Turbines Siemens has implemented AI in its gas turbine factories to optimize performance and reduce emissions. The AI system analyzes sensor data from hundreds of turbines to predict optimal operating parameters, resulting in significant efficiency improvements and reduced environmental impact.
Future Trends
- Lights-Out Manufacturing: Fully automated “lights-out” factories, where production can run without human presence.
- Digital Twins: The use of AI-powered digital twins (virtual replicas of physical systems) for simulation and optimization is likely to increase.
Retail and E-commerce
AI is transforming the retail landscape, both online and in physical stores, by personalizing customer experiences and optimizing operations.
Key Applications
- Personalized Recommendations: AI algorithms analyze customer data to provide personalized product recommendations.
- Inventory Management: AI can predict demand and optimize inventory levels across complex supply chains.
- Dynamic Pricing: AI systems can adjust prices in real-time based on various factors like demand, competitor pricing, and inventory levels.
Case Study: Amazon’s AI-Powered Recommendation Engine Amazon’s recommendation system, powered by machine learning, is responsible for a significant portion of the company’s sales. The system analyzes a user’s browsing and purchase history, as well as the behavior of similar customers, to provide highly relevant product recommendations.
Future Trends
- Augmented Reality Shopping: We anticipate increased use of AI-powered augmented reality for virtual try-ons and product visualization.
- Autonomous Stores: Following Amazon Go’s lead, more retailers are likely to experiment with AI-powered, cashier-less stores.
Transportation and Logistics
AI is driving significant changes in transportation and logistics, from route optimization to autonomous vehicles.
Key Applications
- Route Optimization: AI can analyze traffic patterns, weather conditions, and other factors to optimize delivery routes.
- Demand Forecasting: Machine learning models can predict shipping demand, allowing for more efficient resource allocation.
- Autonomous Vehicles: While still in development, AI is at the heart of self-driving car technology.
Case Study: UPS’s ORION System UPS has implemented an AI-powered system called ORION (On-Road Integrated Optimization and Navigation) to optimize delivery routes. The system analyzes data from millions of packages and thousands of routes to find the most efficient delivery paths, saving the company millions of gallons of fuel annually.
Future Trends
- Drone Deliveries: AI-powered drones for last-mile delivery are likely to become more prevalent, particularly in rural or hard-to-reach areas.
- Predictive Logistics: We expect to see more sophisticated AI models for predicting and mitigating supply chain disruptions.
Energy and Utilities
AI is playing an increasingly important role in the energy sector, particularly in the transition to renewable energy sources and smart grid management.
Key Applications
- Energy Forecasting: AI can predict energy demand and supply, crucial for balancing grids with intermittent renewable sources.
- Smart Grid Management: AI algorithms can optimize the distribution of electricity across smart grids.
- Predictive Maintenance: Similar to manufacturing, AI can predict when energy infrastructure needs maintenance.
Case Study: DeepMind and Google’s Data Center Cooling Google used DeepMind’s AI to reduce the energy used for cooling its data centers by 40%. The AI system analyzes data from thousands of sensors and makes real-time adjustments to optimize cooling efficiency.
Future Trends
- AI in Renewable Energy: We anticipate increased use of AI for optimizing renewable energy systems, from solar panel positioning to wind farm layout.
- Energy Storage Optimization: AI is likely to play a crucial role in managing and optimizing energy storage systems, critical for the wider adoption of renewable energy.
These industry-specific applications demonstrate the wide-ranging impact of AI across different sectors. As AI technology continues to evolve, we can expect to see even more innovative use cases emerge, further transforming these industries and creating new opportunities for value creation.
The Future of Enterprise AI – Emerging Trends and Preparing for What’s Next
As we look towards the horizon of AI in the enterprise, several emerging trends and technologies are poised to reshape the landscape of business and society.
Emerging Trends and Technologies to Watch
1. Artificial General Intelligence (AGI) – While current AI systems are designed for specific tasks, the essence grail of AI research is Artificial General Intelligence – AI that can perform any intellectual task that a human can.
Current State: AGI remains a theoretical concept, with no systems yet approaching human-level general intelligence.
Future Potential: If achieved, AGI could revolutionize every aspect of business and society, potentially solving complex global challenges and accelerating innovation across all fields.
2. Quantum AI – The intersection of quantum computing and AI holds immense potential for solving complex problems beyond the capabilities of classical computers.
Current State: Quantum AI is in its infancy, with researchers exploring how quantum algorithms could enhance machine learning.
Future Potential: Quantum AI could dramatically accelerate drug discovery, optimize complex systems like global supply chains, and potentially break current encryption methods.
3. Neuromorphic Computing – This emerging field aims to create AI hardware that mimics the structure and function of biological neural networks.
Current State: Companies like Intel and IBM are developing neuromorphic chips, but they’re not yet widely deployed in commercial applications.
Future Potential: Neuromorphic computing could lead to AI systems that are more energy-efficient, faster at learning, and better at handling uncertainty and noise in data.
4. AI-Human Symbiosis – The future of AI is not just about autonomous systems, but also about creating seamless interactions between humans and AI.
Current State: We’re seeing early examples of this in AI assistants and augmented reality applications.
Future Potential: Future AI systems might act as cognitive extensions of human intelligence, enhancing our decision-making, creativity, and problem-solving capabilities.
5. Federated Learning and Privacy – Preserving AI As data privacy concerns grow, there’s increasing interest in AI techniques that can learn from distributed datasets without compromising individual privacy.
Current State: Companies like Google are already implementing federated learning in products like Gboard.
Future Potential: These techniques could allow for the development of powerful AI models in sensitive domains like healthcare, without the need to centralize personal data.
Predictions for AI’s Long-term Impact on Business and Society
- Hyper-Personalization at Scale – AI will enable businesses to offer unprecedented levels of personalization in products and services, tailoring experiences to individual preferences and needs at a massive scale.
- Autonomous Organizations – We may see the emergence of Decentralized Autonomous Organizations (DAOs) that operate entirely through AI-driven smart contracts, potentially reshaping corporate structures and governance.
- AI-Driven Scientific Discovery – AI could accelerate scientific research, potentially leading to breakthroughs in fields like materials science, drug discovery, and clean energy technology.
- Transformed Labor Markets – The nature of work will continue to evolve, with AI automation leading to the decline of some job categories but also the creation of entirely new roles and industries.
- Enhanced Human Capabilities – AI could augment human cognitive abilities, potentially leading to significant advancements in fields like education, creativity, and problem-solving.
Preparing for an AI-Driven Future
Cultivate a Culture of Continuous Learning – Organizations should foster a culture where continuous learning is not just encouraged but expected. This could involve;
- Implementing AI literacy programs for all employees
- Offering ongoing training and development opportunities in AI-related skills
- Encouraging cross-functional knowledge sharing and collaboration
Develop Ethical AI Frameworks – As AI becomes more pervasive, having robust ethical guidelines will be crucial. Organizations should;
- Establish clear principles for responsible AI development and use
- Implement processes for ongoing ethical review of AI projects
- Engage with stakeholders and the public on AI ethics issues
Invest in Adaptable Infrastructure – To stay ahead of rapid technological changes, organizations should;
- Adopt cloud-based and modular AI architectures that can easily incorporate new technologies
- Implement strong data governance practices to ensure high-quality, ethically sourced data for AI training
- Explore edge computing and technologies to support real-time AI applications
Foster Human-AI Collaboration – Rather than focusing solely on automation, organizations should explore ways for humans and AI to work together synergistically;
- Design AI systems that augment human capabilities rather than replace them
- Develop intuitive interfaces for human-AI interaction
- Train employees on how to effectively work alongside AI systems
Engage in Collaborative AI Research – To stay at the forefront of AI innovation, organizations should;
- Participate in AI research partnerships with academia and industry consortia
- Contribute to open-source AI projects
- Engage in responsible information sharing to accelerate AI progress while managing risks
Prepare for AI Regulation – As governments worldwide grapple with the implications of AI, organizations should;
- Stay informed about emerging AI regulations and standards
- Participate in policy discussions and provide input to regulators
- Implement governance structures that can adapt to evolving regulatory requirements
Conclusion
The future of enterprise AI is both exciting and challenging. While the potential benefits are enormous, realizing them will require careful planning, ethical considerations, and a commitment to continuous learning and adaptation.
Organizations that successfully navigate this landscape will be those that not only leverage AI’s capabilities but also thoughtfully consider its broader implications. They will be the ones that use AI not just to automate and optimize, but to augment human capabilities, create new value propositions, and contribute to solving global challenges.
As we stand on the brink of this AI-driven future, one thing is clear: the most successful enterprises will be those that view AI not as a mere tool, but as a fundamental shift in how we approach problem-solving, decision-making, and value creation.