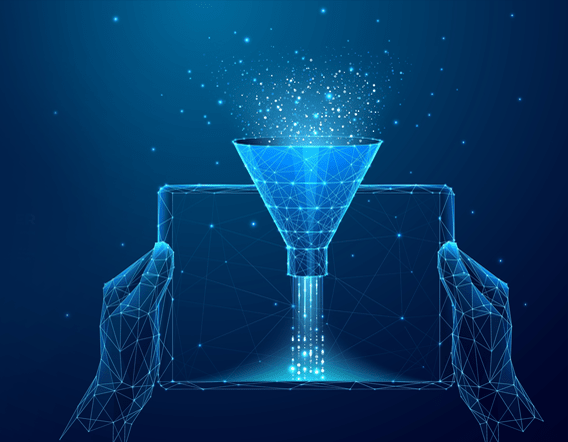
By Alen Alosious
26th Sept, 2024
In today’s data-driven business landscape, the ability to turn raw information into actionable insights is more critical than ever. Enter the concept of “Data Products” – a game-changing approach that’s transforming how businesses leverage data for strategic decision-making and innovation. We’ll delve into the transformative journey from data collection to strategic action, highlighting the pivotal role of these cutting-edge technologies.
The Evolution of Data Management
To understand the current state of data-driven decision-making, it’s essential to trace the evolution of data management;
- Traditional Data Warehousing – Centralized repositories for storing structured data.
- Big Data Era – Emergence of technologies to handle vast volumes of diverse data.
- Data Lakes – Flexible storage solutions for both structured and unstructured data.
- Data Mesh Architecture – Decentralized, domain-oriented approach to data management.
- Data Products – Curated, reusable data assets designed for specific business outcomes.
This evolution has led us to the current paradigm where data is not just stored but actively shaped into valuable assets that drive business decisions.
Understanding Data Products
Data products are the cornerstone of modern data strategies. They are curated collections of high-quality, reusable data assets designed to solve specific business problems or support decision-making processes. They go beyond raw data, incorporating business logic, metadata, and domain knowledge to create actionable insights that can be easily consumed by both human users and AI systems.
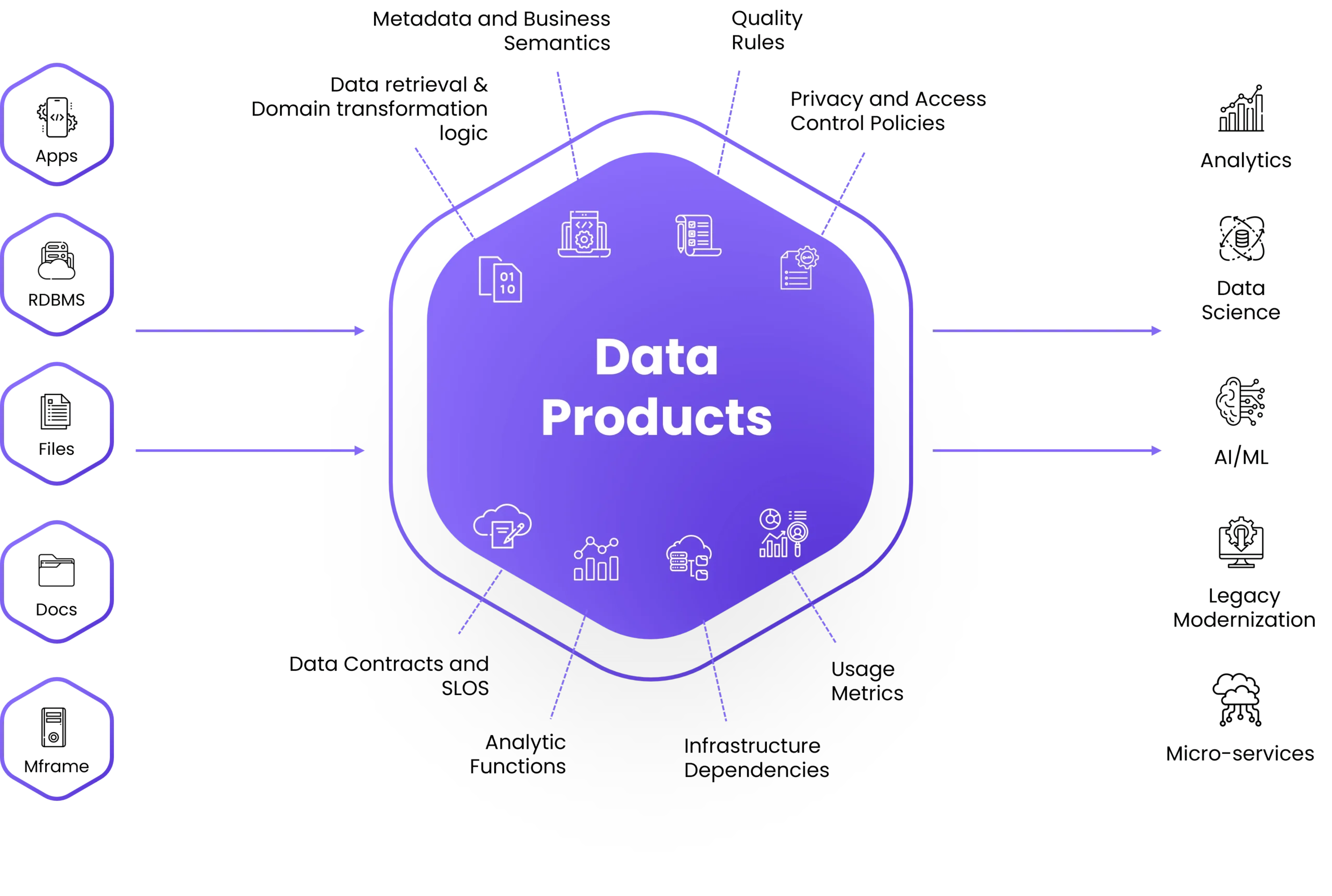
Key Characteristics of Data Products
- Inherent Value – Valuable in themselves, even before specific applications are defined.
- Business Impact – Designed with clear use cases and potential outcomes in mind.
- Discoverability – Easily findable and accessible to intended users.
- Understandability – Clear, well-labeled, and unambiguous.
- Addressable – Consistently located and retrievable.
- Trusted and Curated – Vetted for quality and relevance.
- Secure – Access is controlled and monitored.
- Product Orientation – Managed with a lifecycle approach, considering user needs and feedback.
The Data Product Lifecycle
Creating and maintaining data products involves a structured approach
- Conceptualization – Identifying business needs and use cases.
- Design – Defining data sources, functionality, and presentation.
- Engineering – Building the technical infrastructure and integrating data sources.
- Deployment – Making the product accessible and documenting its use.
- Marketing – Promoting usage and gathering user feedback.
- Usage – Monitoring interactions and performance.
- Maintenance – Continuous improvement based on feedback and evolving needs.
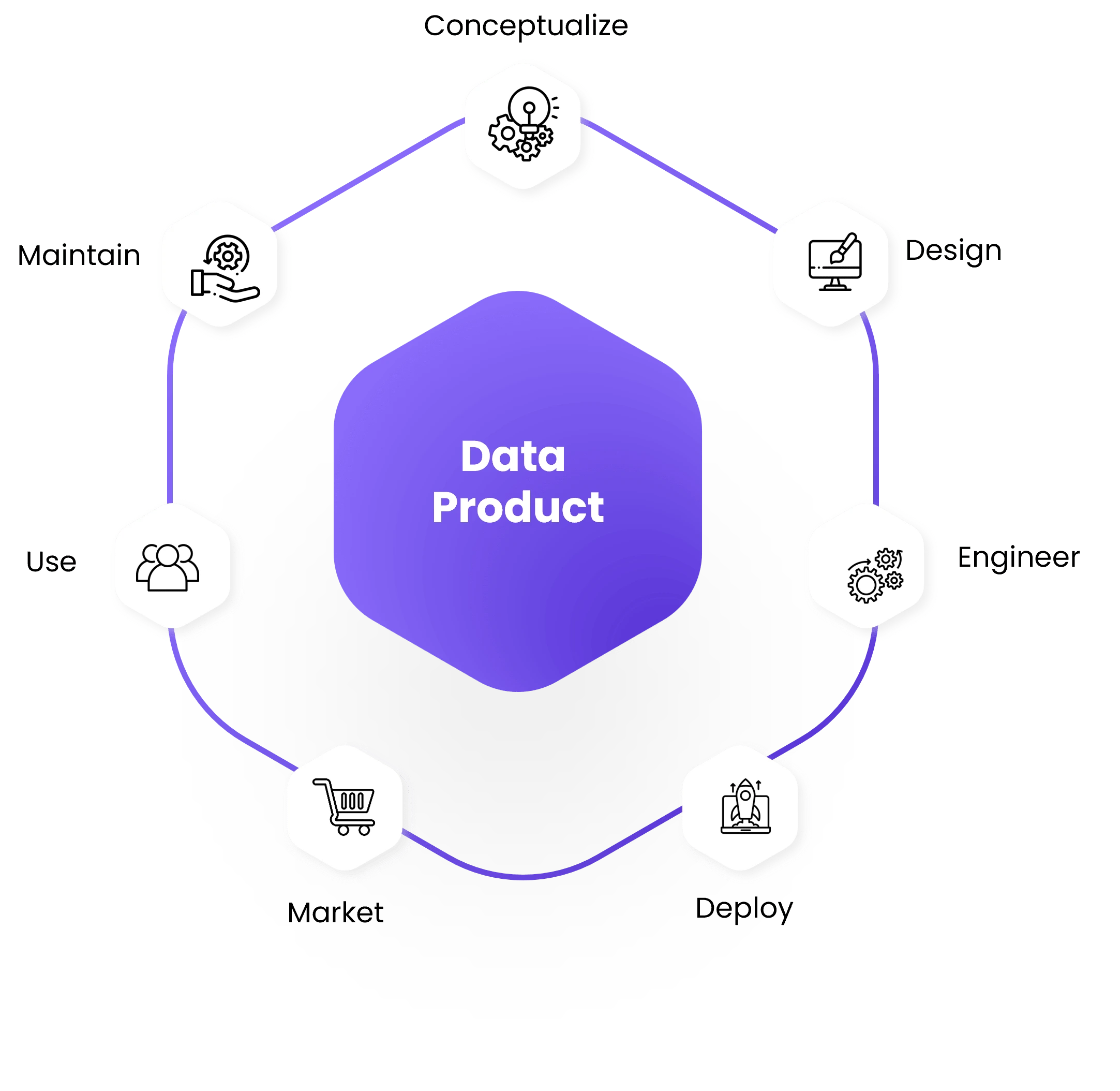
Components of Data Products
Data products serve as a critical bridge between data producers and consumers, revolutionizing how organizations leverage their information assets. According to the Harvard Business Review, companies adopting the data product approach can accelerate the implementation of new use cases by up to 90%, highlighting the transformative potential of this strategy.
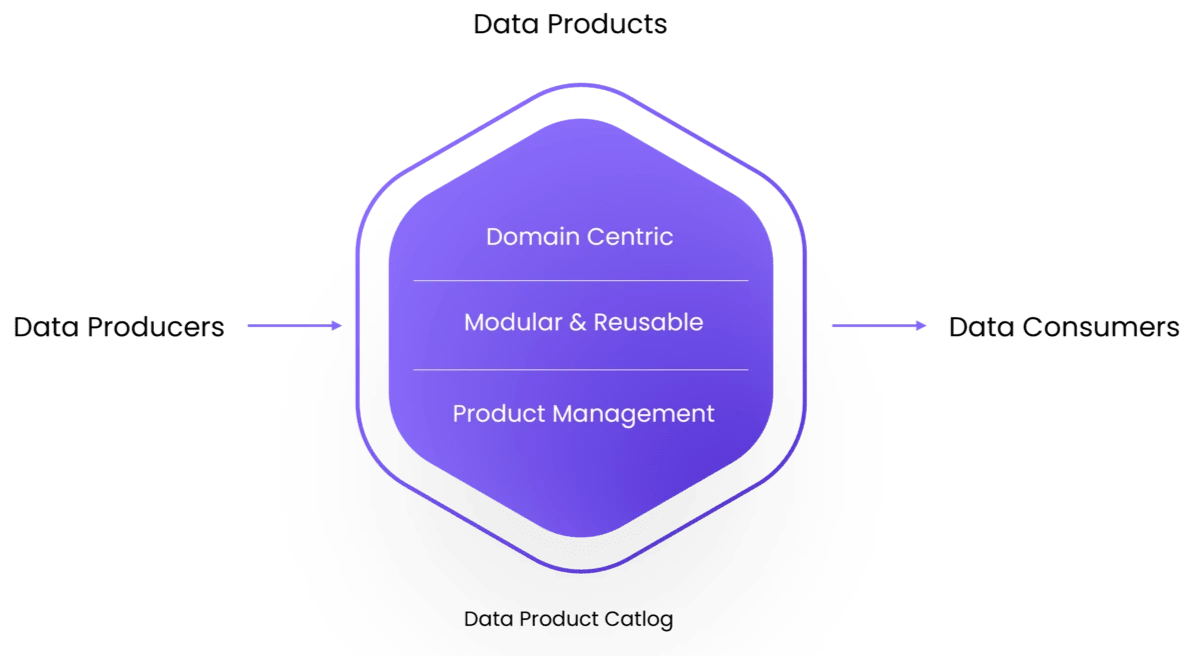
Data Producers – These are the teams or systems that work with various data sets, which can take several forms;
Producers manage the published data model, schema, and business-approved metadata. Importantly, the data they work with often comes from multiple sources and can be either raw or transformed.
- Tables or views in databases
- Machine Learning models
- Streaming data sources
Data Consumers – These are the end-users who interact with data products. They access data through;
- Self-service visualization tools
- APIs for programmatic access
- SQL queries for direct data manipulation
- Other secure access methods
This ecosystem ensures that data is not just stored but actively shaped and delivered in ways that drive business value.
Data products are designed with several crucial characteristics that set them apart from traditional data management approaches;
Domain Centric – The domain model is at the heart of data products, enhancing understanding and providing business context. It serves several critical functions;
- Houses the business logic for data transformations
- Contains analytics calculations and metrics
- Incorporates machine learning models
- Acts as a semantic layer, presenting business-friendly domain data and insights
- Abstracts away technical complexities, making data more accessible to non-technical users
Modular and Reusable – Data products are built with modularity in mind. This approach offers several advantages;
- Once created, they can be reused across multiple use cases
- New data products can be built by combining existing ones
- This modular nature accelerates development and ensures consistency across the organization
Product Management Principles – Each data product is managed like a software product;
- Owned and managed by a domain-aligned data team
- The team is responsible for delivering value, satisfying users, and growing the user base
- Lifecycle management ensures the product evolves over time
- Versions and enhancements are based on customer feedback, ensuring continuous improvement
Data Products Catalog – A foundational component of the data product ecosystem;
- Serves as a centralized marketplace for data products
- Enables data producers and consumers to “speak the same language”
- Provides detailed information about each data product;
- Purpose and intended use cases
- Data sources and lineage
- Processing methods and transformations
- Intended audience and access controls
- Facilitates discovery and understanding of available data assets
The Rise of Generative AI
Generative AI, a subset of artificial intelligence capable of creating new content, has emerged as a game-changer in data management and decision-making processes. Unlike traditional AI models that primarily analyze existing data, generative AI can produce new data, text, images, and even code, opening up unprecedented possibilities for data-driven insights.
How Generative AI Enhances Data Products
- Automated Data Enhancement – Generating synthetic data to fill gaps or augment existing datasets.
- Intelligent Data Cataloging – Creating detailed metadata and descriptions for data assets.
- Natural Language Interfaces – Enabling users to interact with data products using conversational queries.
- Predictive Analytics – Generating forecasts and scenarios based on historical data patterns.
- Content Generation – Creating reports, summaries, and visualizations from raw data.
From Data to Decisions – The Synergy of Generative AI and Data Products
The combination of data products and generative AI is transforming how organizations move from raw data to actionable decisions. Let’s explore this process;
1. Data Acquisition and Preparation
Traditional Approach – Manual data collection, cleaning, and integration.
Enhanced Approach with Gen AI and Data Products
- Generative AI analyzes existing data sources, automatically generating metadata and tagging systems.
- AI-powered data products suggest new data sources to fill gaps in current datasets.
- Automated data quality checks and anomaly detection ensure high-quality inputs.
Real-world Impact: A global pharmaceutical company implemented this approach, resulting in:
- 40% reduction in data preparation time
- 25% improvement in data completeness
- Identification of 3 previously overlooked data sources crucial for commercial business success
2. Data Transformation and Modeling
Traditional Approach – Manual coding for ETL processes and model development.
Enhanced Approach
- Generative AI creates code for data ingestion, transformation, and orchestration across various platforms.
- AI-powered data products automatically suggest optimal data models based on use cases.
- Continuous learning improves transformation processes over time.
Real-world Impact: A financial services firm adopted this method, achieving;
- 60% reduction in code development time
- 35% improvement in model accuracy
- 50% decrease in time-to-market for new data products
3. Insight Generation and Analysis
Traditional Approach – Manual data exploration and report creation.
Enhanced Approach
- Generative AI powers natural language interfaces for data querying.
- AI-driven data products automatically generate insights and anomaly alerts.
- Advanced visualization techniques create intuitive, interactive dashboards.
Real-world Impact: A retail company leveraged this approach, resulting in;
- 70% increase in data product usage across departments
- 30% improvement in inventory management accuracy
- $15 million annual savings through optimized supply chain decisions
4. Decision Support and Automation
Traditional Approach – Human-led decision-making based on static reports.
Enhanced Approach
- Generative AI simulates various scenarios to support strategic planning.
- AI-powered data products provide real-time recommendations for operational decisions.
- Automated decision-making for low-risk, high-volume processes.
Real-world Impact: A manufacturing company implemented this system, achieving;
- 25% reduction in production downtime
- 20% improvement in overall equipment effectiveness
- 15% increase in profit margins through optimized pricing strategies
Challenges and Considerations
While the combination of generative AI and data products offers immense potential, organizations must navigate several challenges;
- Data Quality and Governance – Ensuring data accuracy and compliance with regulations like GDPR and CCPA.
- Ethical AI Use – Addressing potential biases in AI-generated insights and decisions.
- Skills Gap – Developing talent capable of working with advanced AI systems and data products.
- Integration Complexity – Seamlessly incorporating AI-driven insights into existing business processes.
- Change Management – Fostering a data-driven culture that embraces AI-assisted decision-making.
Conclusion
The synergy between generative AI and data products is ushering in a new era of data-driven decision-making. By transforming raw data into actionable insights, organizations can make faster, more accurate decisions that drive innovation and competitive advantage.
As we’ve seen through various real-world examples, the impact spans across industries, from healthcare and pharmaceuticals to finance and manufacturing. The ability to rapidly generate insights, automate processes, and support complex decision-making is revolutionizing how businesses operate.
However, success in this new paradigm requires more than just technology adoption. Organizations must foster a data-driven culture, invest in skills development, and carefully navigate ethical considerations. Those that can successfully blend the power of generative AI with well-designed data products will be best positioned to thrive in an increasingly complex and data-rich business environment.
The journey from data to decisions is becoming more streamlined, intelligent, and impactful than ever before. As we continue to push the boundaries of what’s possible with AI and data products, one thing is clear: the future belongs to those who can harness the power of information to drive meaningful action.